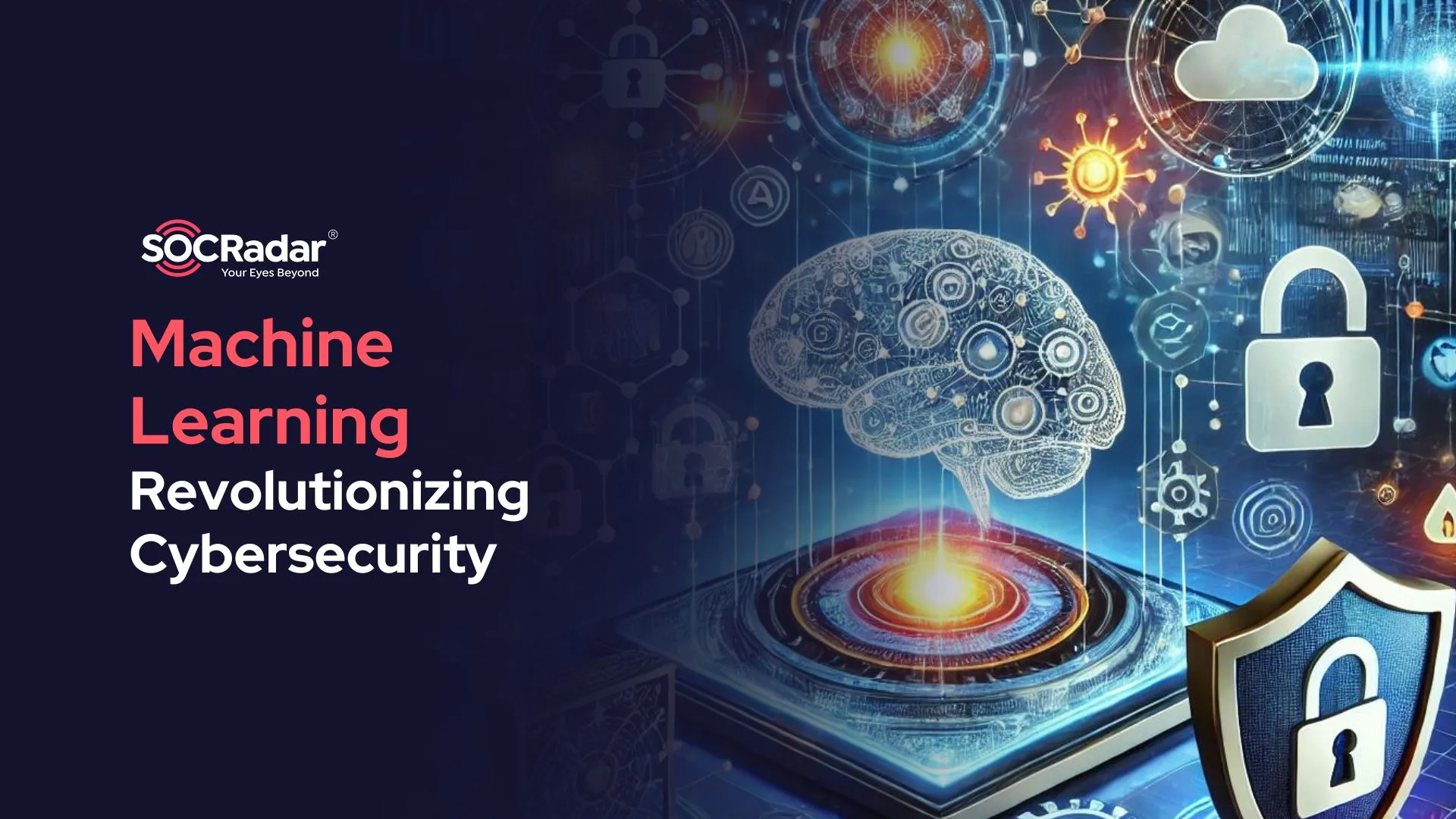
How Machine Learning is Revolutionizing Cybersecurity
In today’s digital age, cyber threats multiply at an alarming rate, putting enormous pressure on organizations to strengthen their defenses. A single breach can have serious consequences, ranging from financial loss and reputational damage to operational disruption and legal liability. `
As threat actors continue to use more sophisticated methods, traditional security measures are often insufficient. This is where Machine Learning (ML), a branch of Artificial Intelligence (AI), comes into play. By learning from large datasets and improving over time, ML enables businesses to detect and mitigate cybersecurity risks at unprecedented speed and accuracy. In this article, we’ll look at how machine learning can transform cybersecurity and look at real-world examples of how it’s used.
The Role of Machine Learning in Cybersecurity
Machine learning is a branch of artificial intelligence that allows systems to learn from data and improve performance without being explicitly programmed. In the context of cybersecurity, this means that ML algorithms can analyze massive amounts of data, identify complex patterns, and make predictions or decisions without human intervention. While AI encompasses natural language processing, computer vision, and other areas, ML is especially well-suited to cybersecurity due to its ability to handle large datasets and adapt to changing threats.
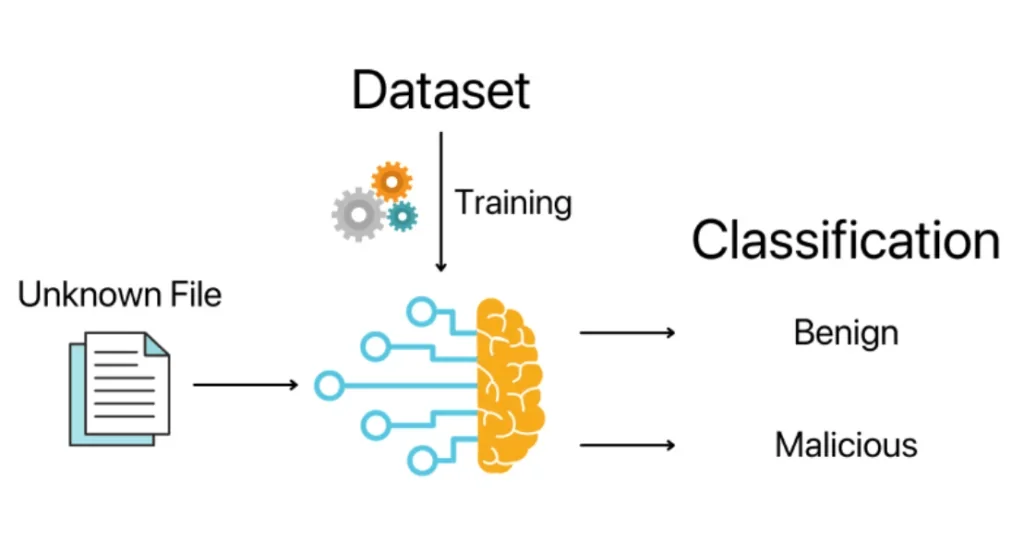
An example use case of machine learning in cybersecurity
Why Machine Learning is Essential for Modern Cybersecurity
Traditional cybersecurity methods frequently rely on predefined rules, such as signature-based detection, which may struggle to keep up with new and evolving threats. Machine learning enables security systems to:
- Predict and Prevent Attacks: By identifying suspicious patterns, algorithms can predict potential threats before they happen.
- Reduce Response Time: ML-powered systems can detect cyberattacks in real time, reducing the time it takes to respond.
- Detect Anomalies: ML can detect even subtle deviations in network behavior, flagging potential insider threats or Advanced Persistent Threats (APTs).
- Automate Monitoring: ML enables continuous monitoring of an organization’s digital assets, with timely alerts for anomalies or vulnerabilities.
These capabilities enable organizations to stay ahead of increasingly sophisticated threat actors, thereby improving overall security posture.
Real-World Applications of Machine Learning in Cybersecurity
Machine learning is being implemented across various sectors to enhance cyber defenses. Here are several real-life examples:
1. Network Traffic Analysis for Threat Detection
Network traffic analysis is one of the most common applications for machine learning in cybersecurity. Machine learning algorithms can analyze massive amounts of network data in real time, assisting in the detection of Distributed Denial of Service (DDoS) attacks and other network-based threats. For example, Darktrace, a cybersecurity firm, employs unsupervised ML to detect anomalies in network traffic, alerting businesses to potential breaches before they escalate.
2. Endpoint Security and Malware Detection
Malware detection is another important area where machine learning excels. Traditional antivirus software detects malware using signatures, but this approach has limitations when dealing with new or evolving malware strains. Machine learning enables systems to analyze file behavior and detect unknown malware. CrowdStrike, for example, employs ML-powered solutions to detect advanced threats, ensuring that endpoints are protected against evolving malware and ransomware.
3. Authentication and Identity Protection
Machine learning is also playing an important role in increasing authentication security. With the rise of sophisticated attacks such as credential stuffing and brute force attacks, organizations are implementing algorithms to detect suspicious login attempts. Companies such as PayPal and Google use ML to monitor login patterns and prevent unauthorized access. Facial recognition and fingerprint scanning rely heavily on its models to ensure secure authentication.
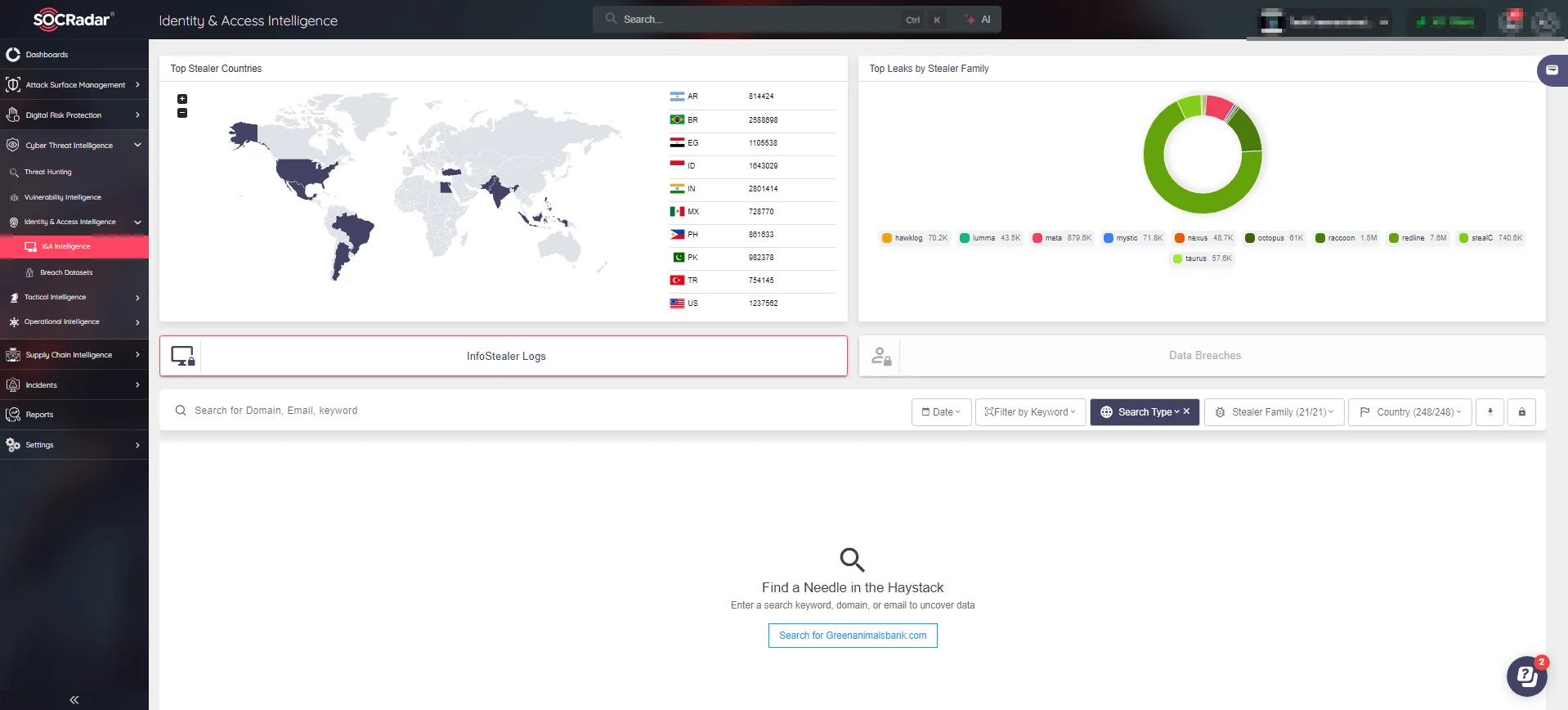
Secure identities and stop unauthorized access with SOCRadar’s Identity & Access Intelligence
4. Fraud Detection in Financial Services
Fraud detection is critical for banks and financial institutions, and machine learning can assist by analyzing transaction data in real time. ML algorithms detect unusual spending patterns and flag possible fraud. Companies such as Mastercard and PayPal use ML to secure millions of transactions per day, protecting both businesses and consumers.
To increase your financial security, SOCRadar’s Fraud Protection monitors dark web marketplaces and carding forums in real time. Powered by AI, this solution actively protects credit card and financial data from fraudsters, sending out timely alerts to help organizations take quick action and avoid financial losses before they occur.
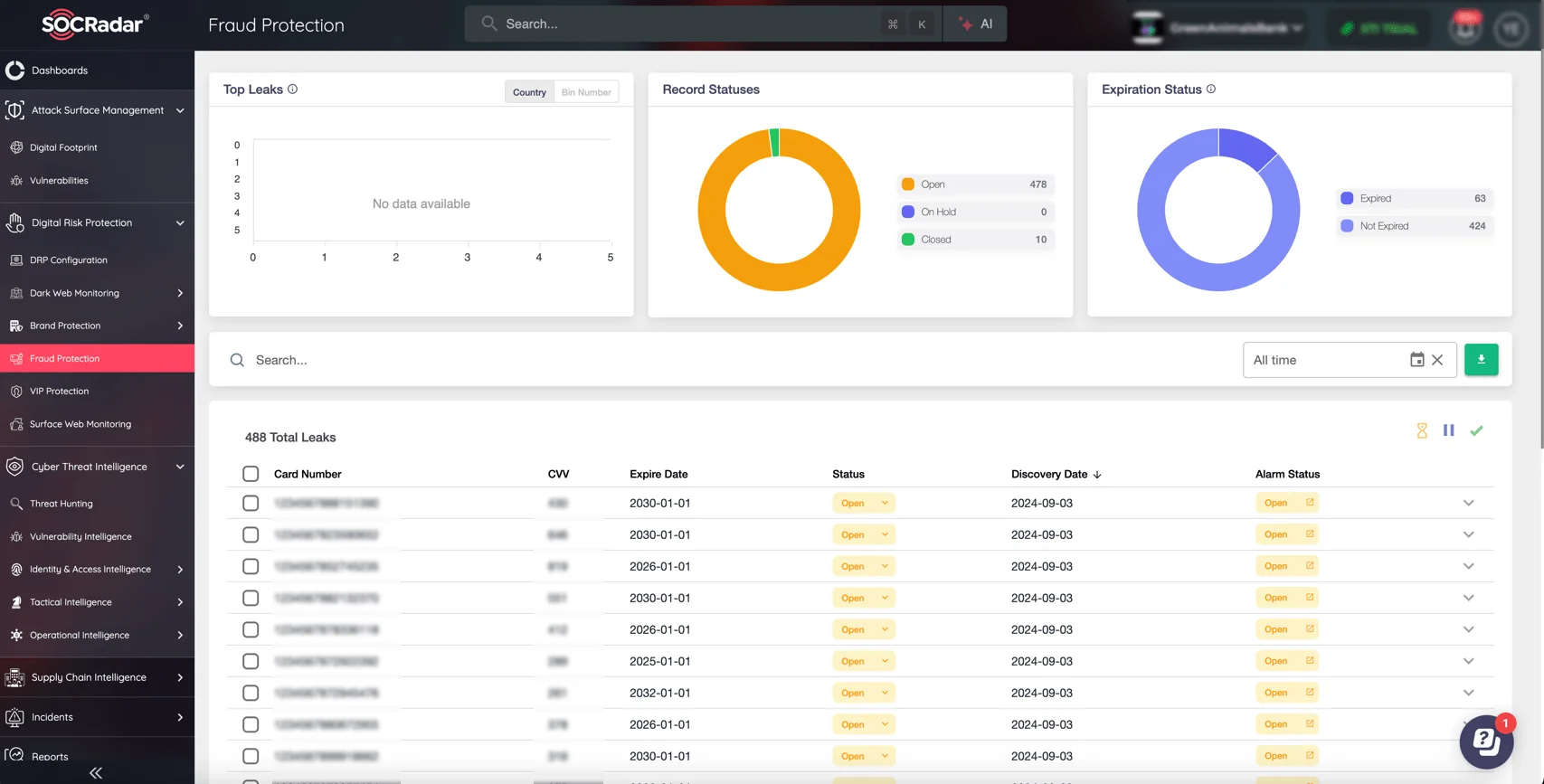
SOCRadar’s Fraud Protection module
5. Attack Surface Management
Organizations’ attack surfaces are constantly expanding in today’s digital landscape as cloud services and remote work arrangements become more common. Monitoring and managing such a large attack surface is difficult, but machine learning provides a solution. SOCRadar, for example, employs machine learning algorithms in its Attack Surface Management (ASM) solution to detect vulnerabilities such as open ports, weak encryption, and out-of-date software in real time. This proactive approach enables companies to mitigate risks before they are exploited by attackers.
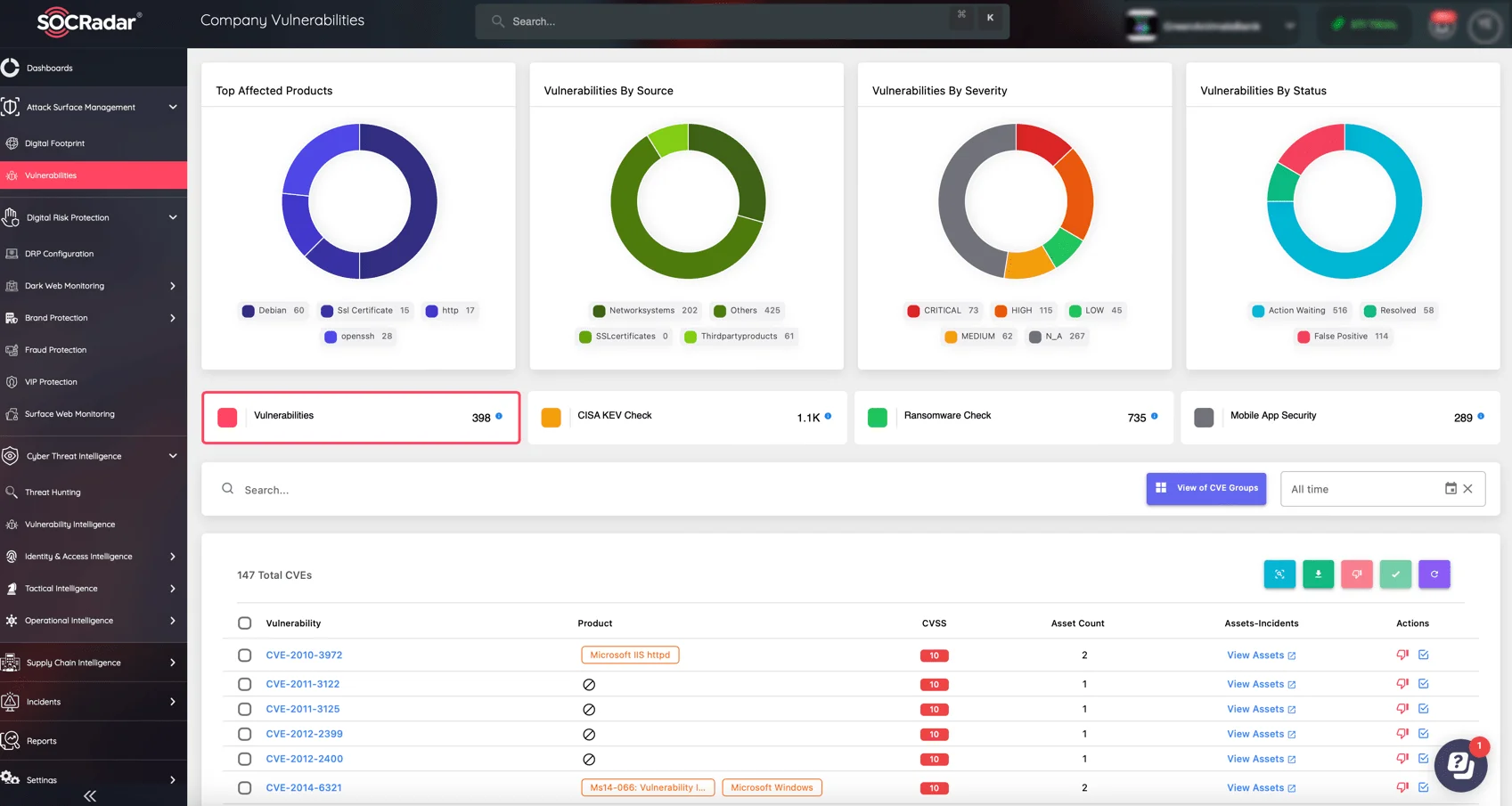
SOCRadar’s Attack Surface Management module
6. Application Security with Machine Learning
Web Application Firewalls (WAFs) are critical for safeguarding web applications against cyberattacks such as SQL injection and Cross-Site Scripting (XSS). Traditionally, WAFs use Application Learning (AL) to create user behavior profiles and send alerts when anomalies are detected. However, AL frequently generates false positives, resulting in unnecessary alerts that overwhelm security teams. Machine Learning (ML) improves WAFs by using statistical models to distinguish between legitimate cyberattacks and benign anomalies, lowering false positives and increasing overall accuracy.
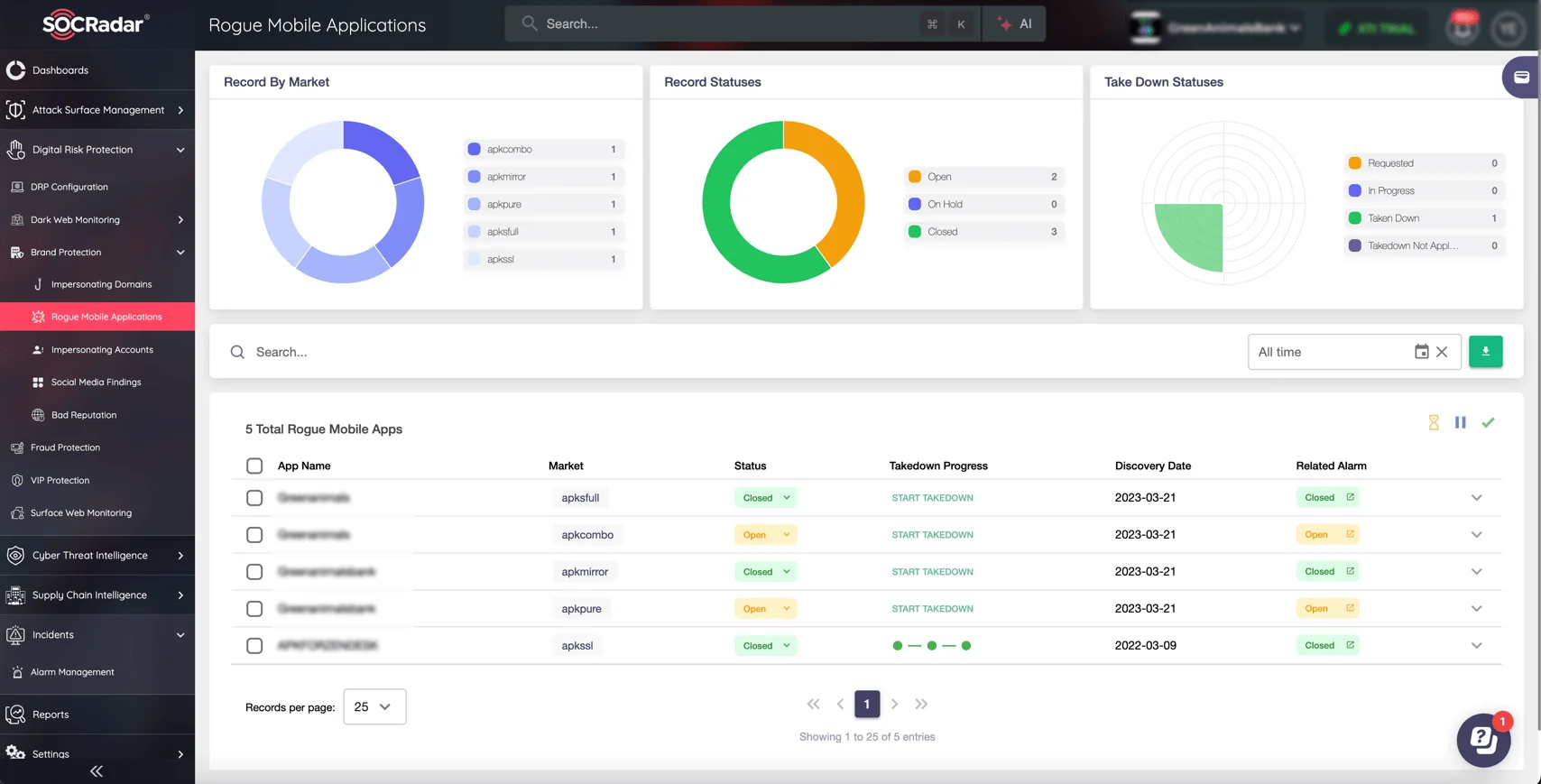
SOCRadar’s Rogue Mobile Applications in Brand Protection
ML-powered WAFs go beyond traditional methods by learning from previous attacks to detect new threats like advanced bots and zero-day vulnerabilities. This reduces the workload for security teams, allowing them to concentrate on real threats while automating tasks such as log analysis and configuration optimization. With these advancements, ML-based WAFs provide stronger, more efficient security for the OSI model’s application layer, allowing organizations to defend against evolving cyber threats while minimizing operational disruption.
The Challenges and Risks of Machine Learning in Cybersecurity
While machine learning offers many advantages, it is not without its challenges. Here are a few key concerns:
- False Positives: While machine learning systems are adept at detecting anomalies, they can sometimes flag legitimate activity as suspicious, leading to unnecessary alerts and wasted resources.
- Data Privacy: Machine learning requires access to vast amounts of data for training, raising concerns about data privacy and security.
- Adversarial Attacks: Threat actors can use machine learning to their advantage by exploiting weaknesses in ML models, manipulating data, or creating malware that can evade detection by ML-based systems.
Additionally, implementing machine learning solutions can be costly, particularly for small and medium-sized enterprises (SMEs). However, with advancements in the technology, more affordable solutions are becoming available.
The Future of Machine Learning in Cybersecurity
As cyber threats continue to evolve, the integration of machine learning into cybersecurity will become even more prevalent. We can expect more advanced algorithms capable of self-learning and adapting to emerging threats. Additionally, ML-powered systems will likely collaborate more with human analysts, improving accuracy while reducing the burden on security teams.
SOCRadar is at the forefront of this transformation, leveraging machine learning in its Brand Protection, Vulnerability Intelligence, and Attack Surface Management services. These solutions offer proactive defense by providing real-time alerts for vulnerabilities, monitoring the dark web for exposed data, and continuously assessing an organization’s attack surface.
For organizations looking to enhance their security posture, combining traditional security measures with machine learning solutions is essential. By adopting advanced ML-based tools, businesses can stay ahead of cyber threats and ensure a more robust defense strategy.
How SOCRadar Leverages AI in Cybersecurity
SOCRadar utilizes AI to enhance its threat intelligence services, delivering highly targeted and relevant insights for its customers. By integrating artificial intelligence into its platform, SOCRadar tailors its threat intelligence to each client’s unique needs—whether they are in a specific industry or geographical location—thereby reducing irrelevant noise and delivering actionable insights. This customized approach allows organizations to focus on critical threats that directly impact their operations rather than sifting through unnecessary information.
In addition to customization, SOCRadar also applies AI to minimize false positives and negatives, two of the most common challenges in cybersecurity. False positives—erroneous alerts about non-existent threats—can overwhelm security teams, while false negatives—missed real threats—pose serious risks to an organization. SOCRadar’s AI-driven approach reduces false alerts by 90%, enabling teams to focus on real and pressing security concerns. This ensures that security resources are effectively allocated and critical threats are addressed promptly, for more information check “How SOCRadar Uses AI” article.
SOCRadar’s AI-enhanced platform provides real-time support and annotation, making it easier for organizations to interpret threat intelligence quickly and take decisive action. This AI-powered feature is essential in today’s fast-moving cybersecurity landscape, where timely response is critical to preventing and mitigating risks. SOCRadar’s suite of services, including Brand Protection, Vulnerability Intelligence, and Attack Surface Management, all benefit from these AI-driven advancements, helping organizations stay ahead of evolving cyber threats.
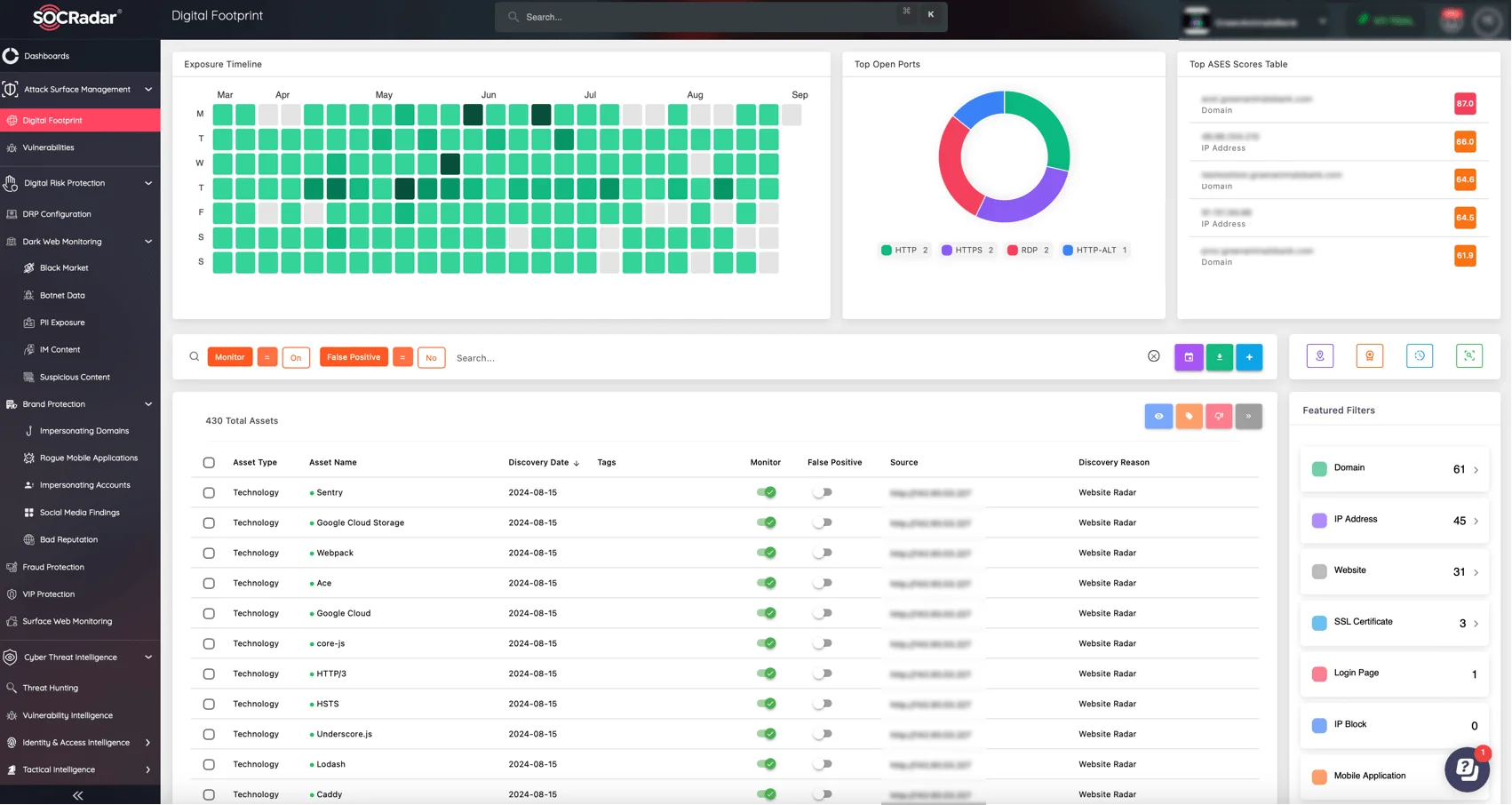
SOCRadar’s Attack Surface Management Digital Footprints
Conclusion
Machine learning is revolutionizing cybersecurity, enabling faster and more accurate detection of threats and automating responses to incidents. From network traffic analysis to fraud detection and attack surface management, it is helping organizations stay one step ahead of threat actors. As technology continues to advance, its role in cybersecurity will only grow, making it a key component of modern defense strategies. SOCRadar’s cutting-edge solutions highlight how machine learning can be effectively integrated into your cybersecurity plan, providing comprehensive protection against today’s most pressing cyber threats.