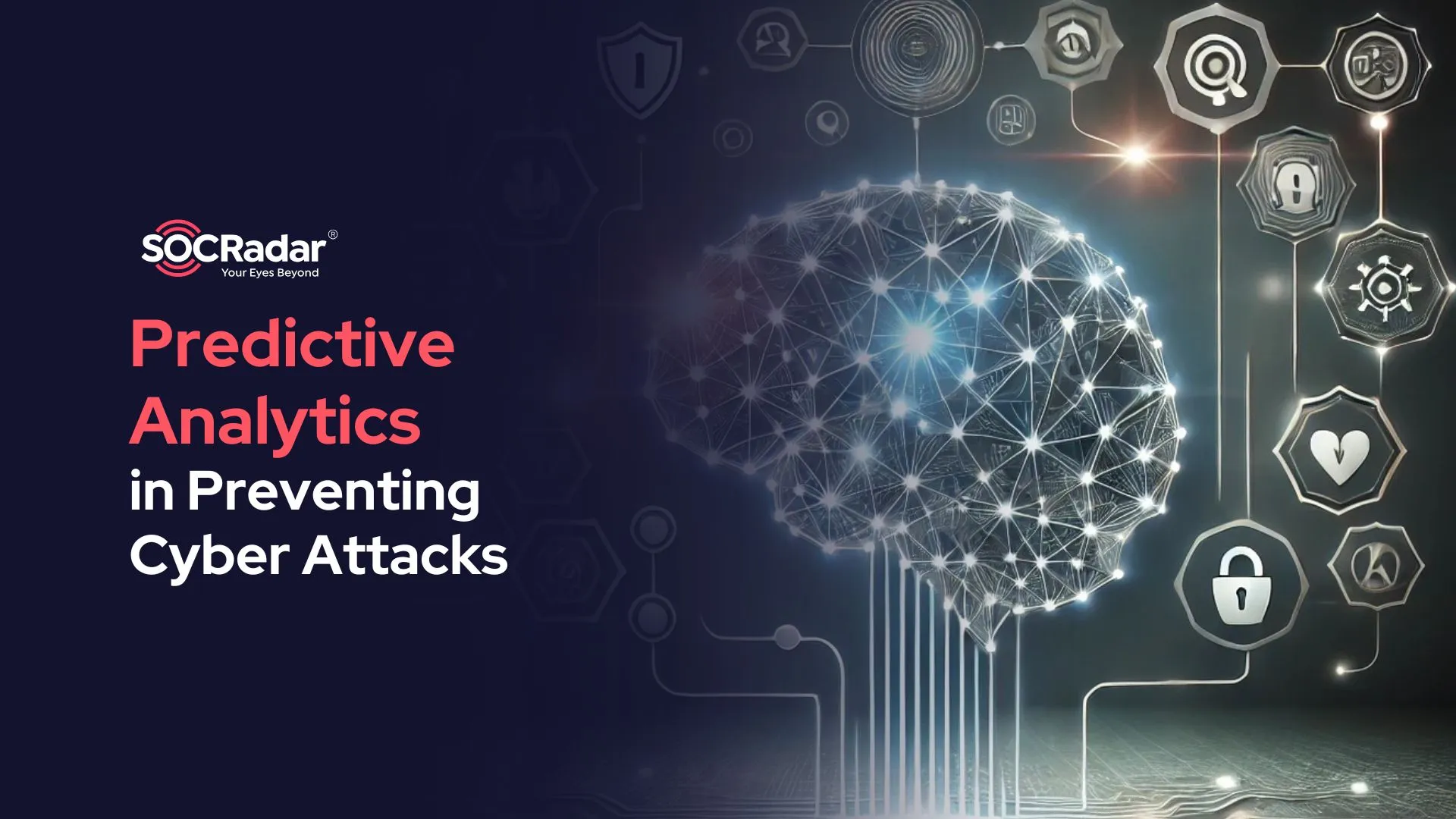
The Role of Predictive Analytics in Preventing Cyber Attacks
Digital threats of today require cybersecurity strategies to anticipate and neutralize cyber attacks before they occur. This is where predictive analytics comes into play—a transformative approach that leverages data to foresee potential cyber threats and vulnerabilities.
Defining Predictive Analytics
Predictive analytics is the practice of analyzing current and historical data to make informed predictions about future events. Unlike traditional analytics, which focuses on understanding past incidents, predictive analytics proactively anticipates potential risks and suggests preventive measures.
Main Steps of Predictive Analytics
To grasp its significance in cybersecurity, it’s crucial to understand the three steps of predictive analytics:
- Data Collection and Preparation
High-quality data is the backbone of predictive analytics. Cybersecurity-related data may include network logs, threat intelligence feeds, user behavior data, and historical attack patterns. - Analysis
Predictive analytics employs algorithms to identify patterns and anomalies within data. Without the analysis of the collected data, predicting the possible futures would be impossible. - Actionable Insights
The ultimate goal is to produce actionable insights. After analyzing the collected data, predictions and insights about the future must be generated in order to take action. These insights empower cybersecurity teams to prioritize resources, preempt vulnerabilities, and address threats in real time.
SOCRadar Threat Hunting Module to detect threats
Predictive Analytics vs. Traditional Cybersecurity Approaches
Traditional cybersecurity methods often rely on reactive measures—responding to incidents after they occur. While tools like firewalls and antivirus software are essential, they fall short in anticipating novel attack methods. Predictive analytics complements these defenses by offering foresight.
How Predictive Analytics Enhances Cybersecurity
In today’s digital ecosystem, cyber threats are becoming more advanced, persistent, and disruptive. Traditional reactive defenses are no longer sufficient to protect organizations against sophisticated attackers. Predictive analytics introduces a proactive approach, equipping cybersecurity teams with tools to identify and neutralize threats before they can cause harm.
SOCRadar’s Free Tools
Predictive analytics transforms vast amounts of cybersecurity data into actionable intelligence. Aggregating and analyzing data from various sources, including threat intelligence feeds, social media, and the dark web, organizations can identify patterns and anomalies, which will help them detect potential threats in their earliest stages.
The following are some examples of how predictive analytics can enhance cybersecurity:
- Early warning systems can identify chatter about new exploits or attack campaigns can help organizations prepare defenses in advance.
- With vulnerability prioritization organizations can evaluate which vulnerabilities are most likely to be targeted based on exploit trends and benefit from smarter patch management.
- By analyzing malware signatures and behaviors, predictive models can flag emerging malware strains based on similar characteristics.
- Machine learning algorithms can analyze email metadata, language patterns, and sender reputation to predict and block phishing attempts.
- Threat actors often disguise their activities to mimic normal user behavior. Predictive analytics excels in analyzing user and entity behavior, identifying subtle deviations that indicate malicious activity.
- Insider threat detection can help with unusual access patterns, such as downloading large volumes of sensitive data and can trigger alerts before significant damage occurs.
- By monitoring login behaviors, suspicious activity, such as logins from unusual locations or devices, can be flagged and can trigger alerts before significant damage occurs.
- Predictive analytics not only identifies potential threats but also can be automated to respond swiftly. This reduces the time needed to detect and neutralize attacks, minimizing potential damage. Predefined actions for certain cases can go under predictive models. By isolating compromised devices or blocking malicious IPs, without manual intervention, organizations can benefit from predictive methods to protect their systems.
Key Techniques in Predictive Cyber Analytics
Predictive analytics relies on a blend of advanced technologies, methods, and techniques to forecast and mitigate cyber threats.
Behavioral Analytics and Anomaly Detection
Behavioral analytics and anomaly detection focus on identifying deviations from normal patterns in users, devices, and networks to detect potential cyber threats. By establishing baselines of typical activity, predictive systems can flag suspicious behaviors that may signal cyber attacks.
User Behavior Analytics (UBA) enables organizations to identify unusual activities, such as logins from unexpected locations or excessive file access. Similarly, Entity Behavior Analytics (EBA) monitors devices and applications to detect anomalies, including rogue software installations or network intrusions.
Outliers often indicate cyber risks, and techniques like clustering, which groups similar data points to isolate irregularities like abnormal traffic patterns, and time-series analysis, which tracks sequential data for unusual spikes or trends, can help identify cases of data exfiltration or unauthorized access.
Predictive Threat Modeling
Predictive threat modeling is a proactive cybersecurity approach that simulates potential attack scenarios using historical data and threat intelligence.
This method helps organizations understand how attackers might exploit vulnerabilities, enabling them to prioritize defensive measures effectively.
Techniques such as attack path analysis, which predicts the sequence of events leading to a potential breach, and risk scoring, which evaluates the likelihood and severity of vulnerabilities to guide mitigation strategies, are key components of this modeling.
Real-Time Data Analysis
Predictive analytics leverages real-time data streaming and analysis to detect threats as they emerge. This approach significantly reduces detection times, enabling companies to quickly identify and mitigate threats, thereby minimizing potential damage.
Discover if your company is mentioned on dark web forums with SOCRadar’s Advanced Dark Web Monitoring
Implementing Predictive Analytics for Attack Prevention
Integrating predictive analytics into your cybersecurity strategy can seem like a daunting task, but with the right approach, it becomes a manageable and transformative process. This chapter outlines the key steps and best practices for implementing predictive analytics to prevent cyber attacks.
Establish Clear Objectives
Before implementing predictive analytics, define your organization’s cybersecurity goals. You can use the following as example objectives:
- Detecting phishing attempts before employees are affected.
- Identifying and mitigating insider threats.
- Predicting vulnerabilities most likely to be exploited.
- Enhancing overall threat intelligence capabilities.
Build a Robust Data Foundation
Data quality and quantity are critical for the success of predictive models.
- Incorporate data from logs, network traffic, endpoint devices, user behavior, and external threat intelligence feeds.
- Regularly validate and clean data to remove duplicates, errors, and irrelevant information.
Employ Predictive Models
The core of predictive analytics lies in developing models tailored to your organization’s unique cybersecurity needs.
- Focus on specific challenges, such as detecting anomalous login behaviors or forecasting DDoS attacks.
- Use the necessary datasets to train your models for those cases.
Automate Threat Detection and Response
Integrating predictive analytics with automated response systems amplifies its effectiveness. You can:
- Configure workflows to isolate compromised systems or block suspicious IP addresses automatically.
- Use predictive models to rank alerts or other necessary information (for example vulnerabilities) based on severity, reducing the noise for security teams.
By aligning predictive analytics with measurable goals, organizations can focus on delivering targeted outcomes. While the examples provided serve as illustrative benchmarks, tailoring them with specific quantifiable targets enables organizations to align these strategies with their unique priorities and operational needs.
Conclusion
Predictive analytics is revolutionizing the field of cybersecurity by providing organizations with a proactive approach to identifying and mitigating threats.
Implementing such a framework requires a strategic roadmap—starting with clear objectives, establishing a robust data foundation, and employing sophisticated predictive models to automate threat detection and response processes.
As cyber threats grow more complex, predictive analytics stands out as a critical tool in the arsenal of modern cybersecurity. It bridges the gap between traditional reactive strategies and forward-looking intelligence, enabling organizations to build resilient defenses and maintain trust in an increasingly interconnected digital world.