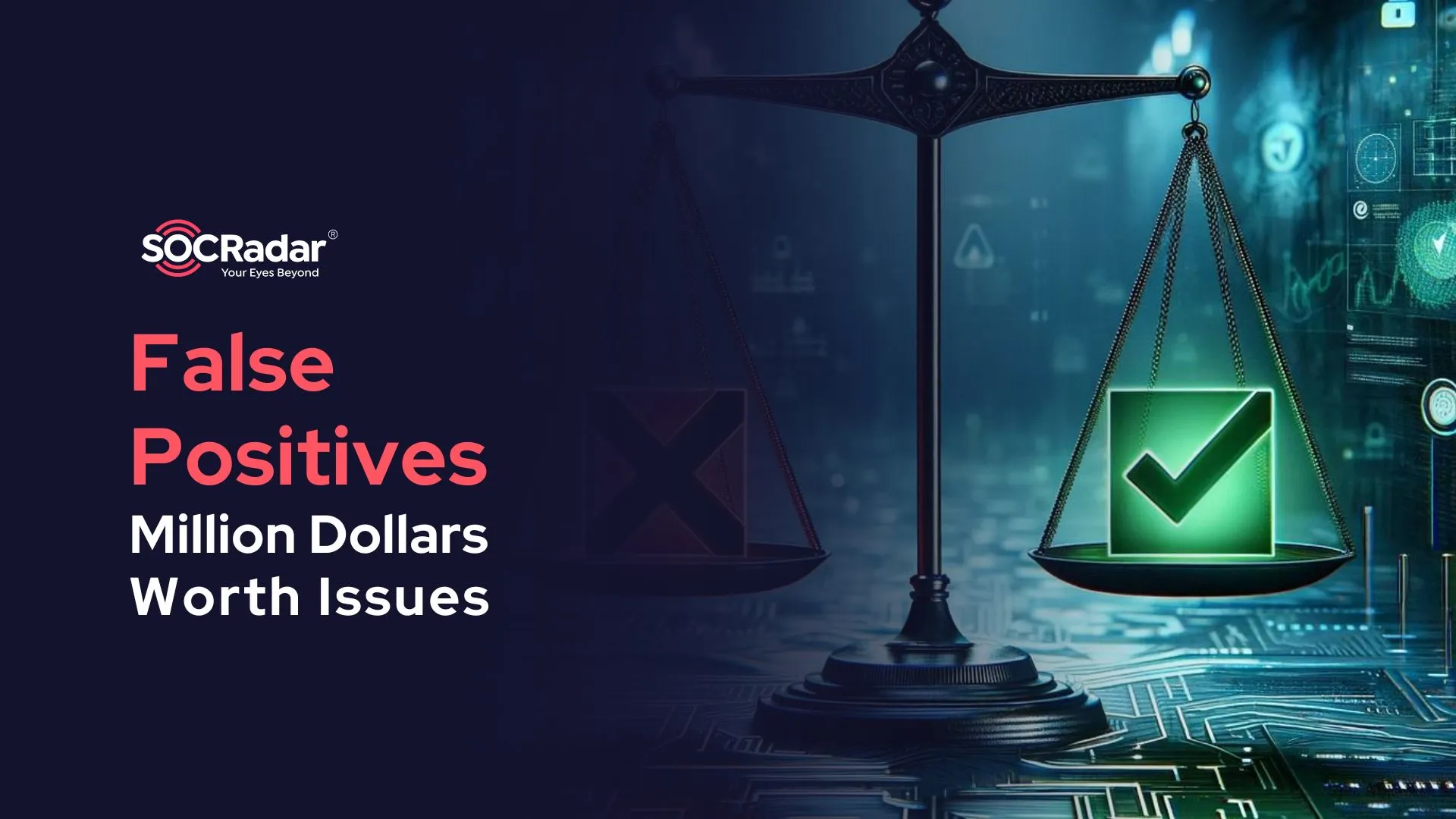
False Positives: Million Dollars Worth Issues – Spoiler: It is not even an issue.
Fairy tales for adults: from the boy who cried wolf to alert fatigue.
Once upon a time, a shepherd boy, bored with his dull life in the pasture, decided to amuse himself by pretending a wolf was attacking his flock. He ran to the village shouting, “Wolf! Wolf!” The villagers rushed to his aid, only to find it was a false alarm and the boy laughing at them. A few days later, he repeated the joke, with the same result.
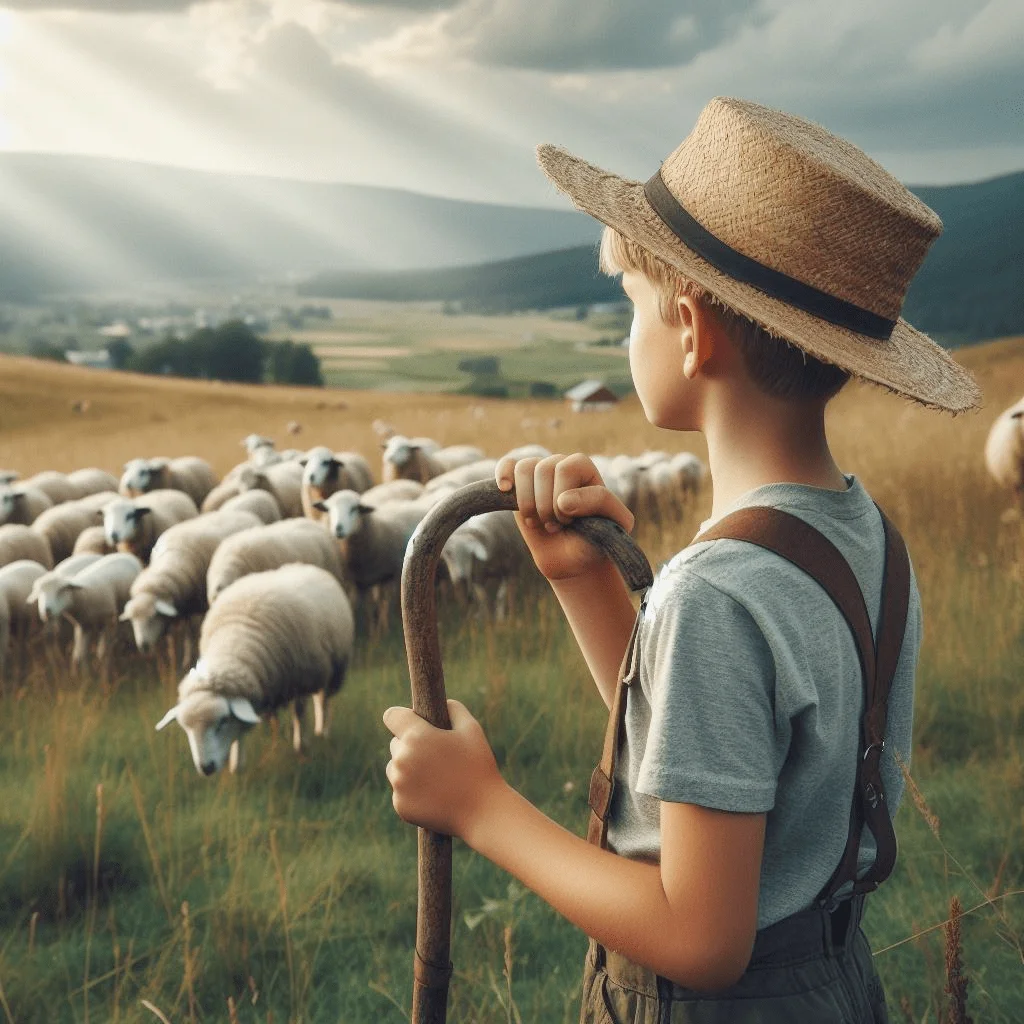
Visual illustrating the story of The Boy Who Cried Wolf (Dall-E)
However, one evening, a real wolf attacked the sheep. The boy ran to the village, shouting desperately for help, but the villagers, thinking it was another joke, ignored him. Tragically, the wolf killed many of the boy’s sheep, and there was no one to help.
This story, found in many cultures, not only teaches the consequences of lying but also illustrates the concept of false positives and the alert fatigue they cause. Unlike the boy’s pranks, false positives in security arise from a lack of detailed information or sufficient confirmation.
The Dangers of False Positives in Modern Industries
From finance to information security, almost every industry grapples with false positives. These erroneous alerts lead to financial and reputational damage, costing organizations tens of thousands of work hours each year.
Many believe tackling false positives is challenging. This is true if one lacks a proper understanding of the targeted or underlying systems, anomalies, or malicious attempts. False positives not only hinder better vulnerability reports and incident reporting, but also disrupt legitimate operations, real customer interactions, and valid transactions.
Origins and Implications of False Positives
The terms “false positives,” “true positives,” “false negatives,” and “true negatives” originate from statistical hypothesis testing and binary classification, commonly used in fields such as medicine, statistics, and security operations. In the context of machine learning, these terms evaluate the accuracy of tests and decisions. Here, we will focus on their significance in information security and incident response.
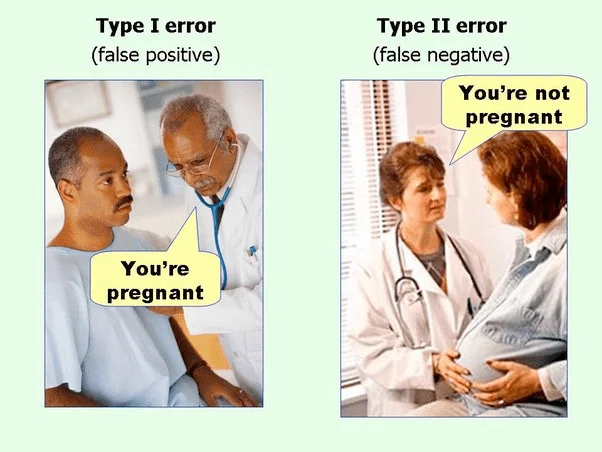
A common visual rotating for years, the source is not obvious.
Understanding the Key Terms
- True Positive (TP): This term refers to a correct positive prediction or result. When your scanning tool reports an SQL Injection vulnerability and you or the tool itself can confirm that the finding is real and the vulnerability exists, this finding can be categorized as a true positive. You or the security tool/service you used reports something, and it is indeed present. You might wonder about the point “as long as I can confirm…”. Here the problem arises: it requires a lot of human resources or technical capability from the tool to confirm the vulnerability’s existence. A smart formula or assumption can be applied in this field: if there is a vulnerability, it should be exploitable. If it is not, it is probably not a vulnerability.
Does this remind you of Aristotle? You are right! Very basic logical rules of human intelligence. Sometimes humans can get confused.
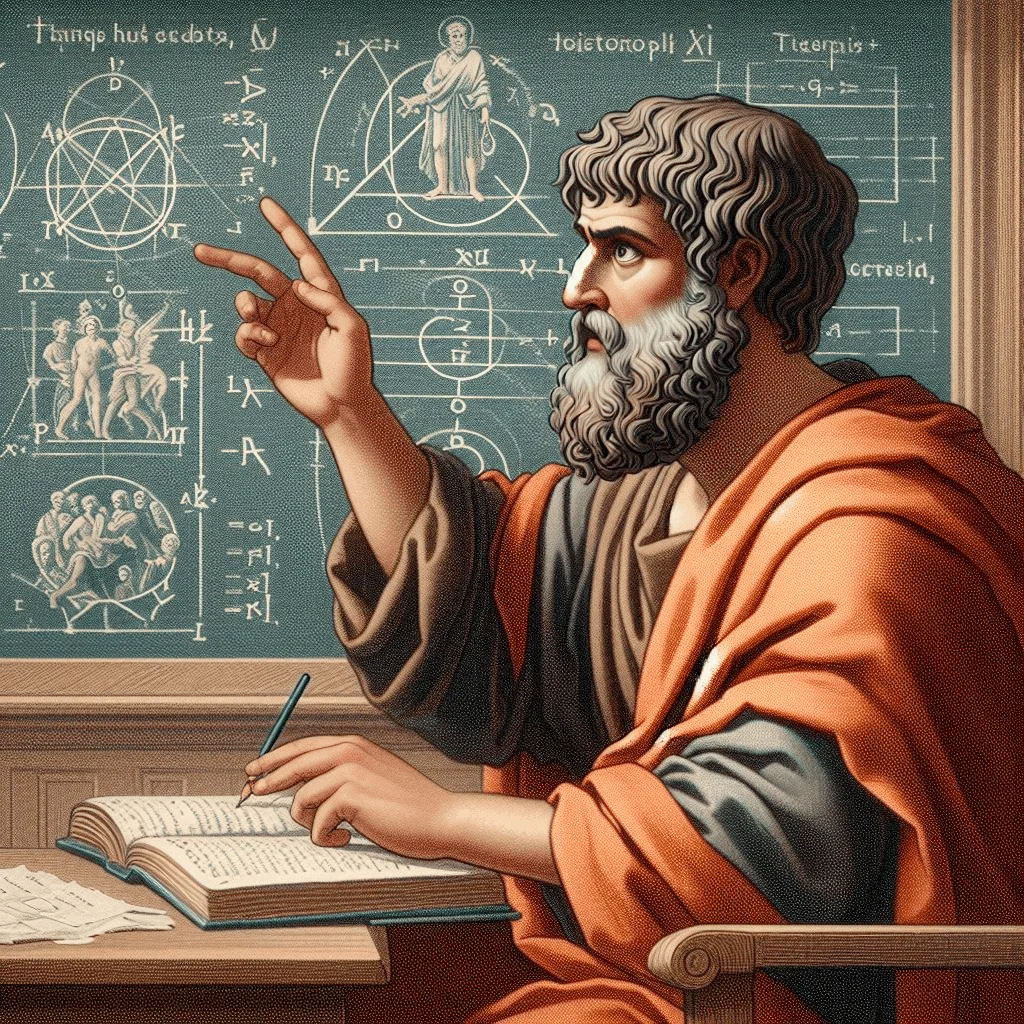
AI-generated visual depicts Aristotle teaching the basic rule of logic, Dall-E
For such a basic logical process, why do many tools and services fail? The answer is simple: to reach this conclusion as a human, you have to spend some time (this is one of the exact problems), and tools and services need to know the internals of the systems very well.
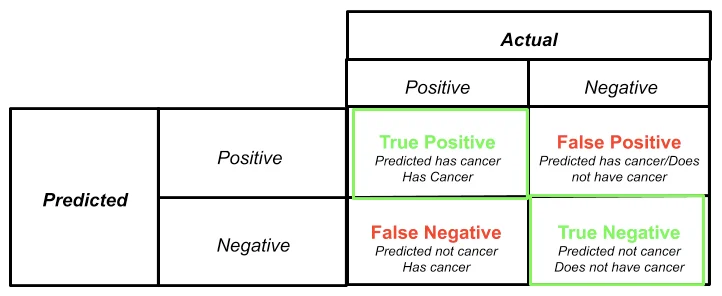
Credit: https://miro.medium.com/v2/resize:fit:1400/1*gr4szbbV3jz3ALlC9BPYTg.png
- True Negative (TN): A true negative represents a correct negative prediction or result. It indicates correctly identifying the absence of a relationship or condition that is truly not present in the data. For example, if you or a tool or service you used, after a security check, did not find an SQL Injection vulnerability on the website, and the website indeed does not have this issue, this finding can be considered a true negative. You assessed a system with a negative mark for the existence of a vulnerability, and it is accurate.
- False Positive (FP): A false positive occurs when a test incorrectly indicates the presence of a condition that is actually not present; where the test wrongly gives a positive result. Let us recall our first example: you or the tool/service you used reported an SQL Injection vulnerability on your website. In reality, this is not true. To increase the severity of the example, let’s say that your website does not have a database connection or the database behind the application is not a relational database. You can easily flag this finding as a false positive. Basically, we are saying that the finding is false.
- False Negative (FN): A false negative is a test result that wrongly indicates the absence of a condition that actually exists. Although false positives can be time-consuming, tiring, and boring, false negatives can be very risky and devastating. Let’s imagine you asked your security staff to scan your new web service for SQL Injection vulnerabilities. After a while, the staff member comes back to you and says they did their best but could not find any SQL Injection vulnerabilities in your web service. However, if it actually has one, marking a finding as “Not Found” or negative can be misleading. This situation can be categorized as a false negative. Again, although false positives require many resources, time, and patience, false negatives, or marking a system as healthy when a vulnerability exists, can be very dangerous.
True Damages of False Positives
False positives can be extremely costly for organizations. Investigations into false positives can consume nearly 10,000 working hours annually, costing around $500,000.
The impact extends beyond information security. In e-commerce, false positives, or false declines, amount to $443 billion in lost revenue annually, far outweighing the actual costs of credit card fraud. Incorrectly blocking legitimate transactions can potentially cost businesses up to $386 billion each year.
Furthermore, false positives damage reputations. They lead to poor customer experiences, with legitimate customers turned away or their transactions blocked. This can result in customer churn, with 38% of consumers stating they would switch merchants after a false decline.
Mitigating False Positives: SOCRadar’s Approach
False positives in security alerts are a significant challenge for security analysts. SOCRadar addresses this issue through several strategies, achieving an 85% success rate in eliminating false positives (FPs) using AI. The remaining alerts are checked and confirmed by SOCRadar’s seasoned experts as part of their professional services. Detailed explanations of the steps to address FPs are:
- Integration with Threat Intelligence: SOCRadar integrates with SIEM (Security Information and Event Management) solutions, which collect logs and data from various sources across the network. This integration enhances SIEM capabilities by providing contextual information about potential threats, allowing analysts to understand attackers’ Tactics, Techniques, and Procedures (TTPs). This reduces false positives and helps analysts focus on critical incidents.
- User-Driven Scoring Approach: SOCRadar prioritizes alerts based on their severity and relevance, assigning scores to ensure analysts focus on the most important threats, thereby reducing noise from false positives.
- Validation and Whitelisting: SOCRadar validates Indicators of Compromise (IoCs) and checks them against a whitelist. With over 43 million whitelist policies, SOCRadar filters out known benign entities, preventing false positives. Analysts can also verify the whitelist status of any IP, hash, or domain.
By implementing these measures, SOCRadar effectively reduces the occurrence of false positives, allowing security teams to operate more efficiently and focus on genuine threats.