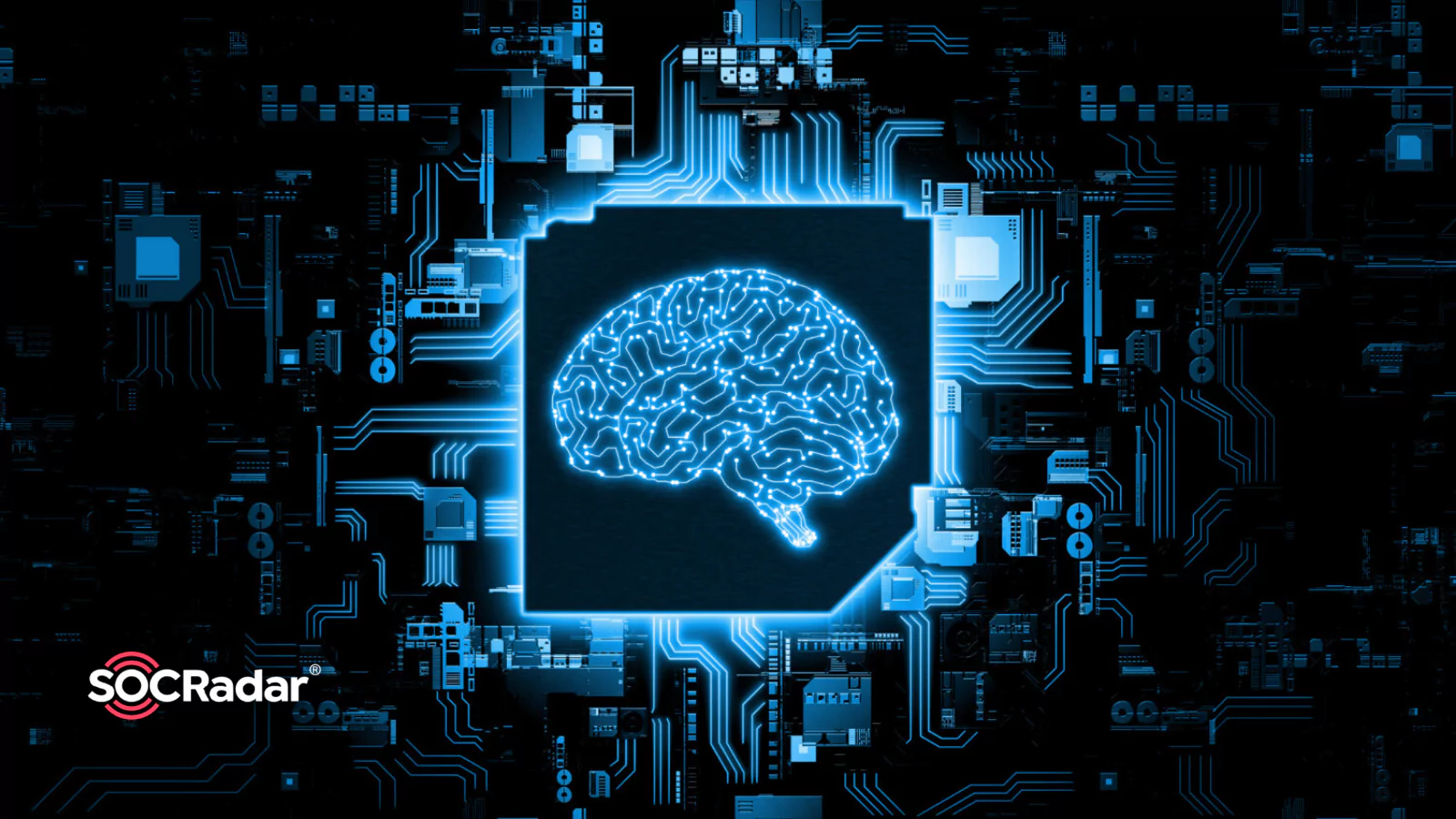
Real-Life Examples of Machine Learning in Cybersecurity
Artificial Intelligence and Machine Learning: A Thriving Concept in the World of Cybersecurity
There’s no question that artificial intelligence and machine learning are two major points of interest in technology in the 21st century. Everyone has heard of the terms, but did you know that they are being actively implemented into cybersecurity solutions?
Before getting into example use cases of machine learning in cybersecurity, it is best to learn what machine learning is and how it differs from AI. Machine learning is, by definition, the implementation of algorithms that can learn through experience and improve its performance automatically, whereas artificial intelligence is the field of creating computer systems capable of mimicking human intelligence.
It can be said that artificial intelligence is a broader term and covers machine learning as a sub-discipline; but in the cybersecurity community, the term machine learning is commonly used rather than artificial intelligence.
Advantages of Applying Machine Learning in Cybersecurity
There are many adversaries in the world with the goal of exploiting vulnerabilities to damage companies, financially or otherwise. A company’s firepower to defend itself may not be enough to evade all of the cyber threats it is facing. That’s where machine learning security solutions come into play. Developing a responsive and evolving system that can learn with every experience can give companies the upper hand against threat actors.
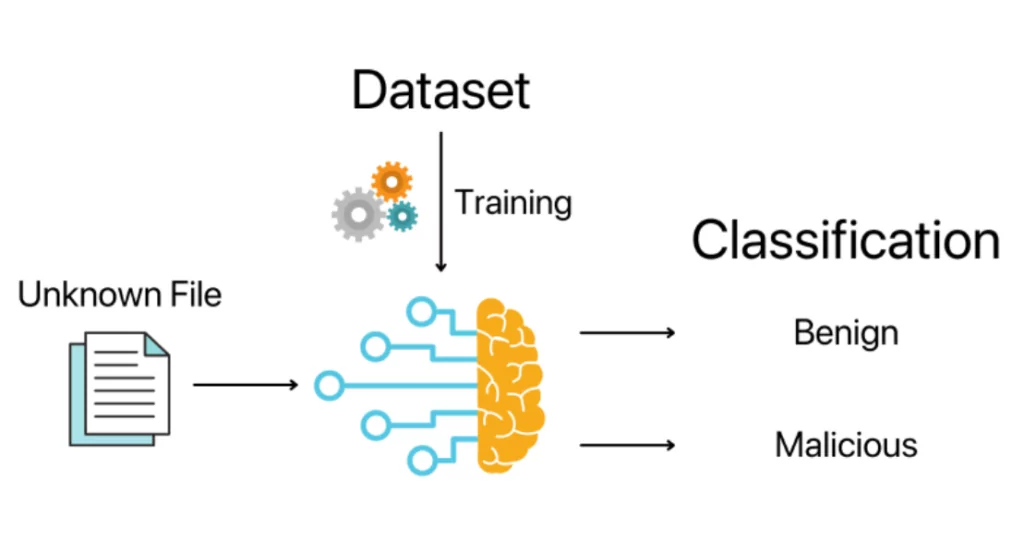
Machine Learning systems can be trained to
- Predict upcoming cyber-attacks and help companies better mitigate the consequences,
- Create alarms and notify companies against cyber threats,
- Rapidly identify cyber-attacks and shorten response time,
- Detect vulnerabilities in the company’s digital structure,
- Continuously monitor the digital attack surface and notify the company against anomalies.
Disadvantages of Machine Learning in Cybersecurity
Even though machine learning algorithms have many advantages and use cases in the cybersecurity sector, we cannot fully trust machine learning to solve all our problems.
The zero-trust architecture should be applied to machine learning as well. No system is without vulnerabilities. Given enough time, every system can be hacked or exploited, so we should treat machine learning systems with caution and take the necessary precautions.
In addition to the security concerns about machine learning systems in cybersecurity, implementing machine learning solutions to cybersecurity problems companies face may be financially costly. Not every company can implement machine learning solutions to their cyber defenses.
Another disadvantage is that adversaries also have access to the science behind AI, which can enable them to find vulnerabilities within existing machine learning solutions to exploit companies. In addition, adversaries can utilize AI and machine learning mindset to develop complex malware that can avoid detection by evolving itself.
Five Real-Life Examples of Machine Learning in Cybersecurity
There are many different use cases of ML in cybersecurity. Below, you can see five main real-life examples selected by analysts at SOCRadar.
1. Network Traffic Analysis
ML algorithms can be implemented within network traffic analysis to detect network-based attacks such as DDoS attacks. For example, a trained algorithm can detect the large volume of traffic a server receives during a DDoS attack and notify authorities within your company for remediation. In addition, the algorithms can also discover the attack vector or the attack type (e.g., TCP Flood), which enables SOC teams to take precautions against upcoming cyber threats in the future.
ML algorithms in network traffic analysis can also identify bots or botnet actions to protect servers against malicious threat actors aiming to exploit servers with botnets.
2. Endpoint Fortification
It is crucial for companies to protect their endpoints against malware and viruses. ML algorithms can fortify endpoint protection and assist anti-viruses and firewalls by identifying unknown cyber threats.
ML algorithms can also classify incoming malicious packages as malware, ransomware, or spyware, helping SOC teams to prioritize and prevent upcoming digital risks.
In addition, ML assisted endpoint protection can also detect anomalies at endpoints and notify authorities, enabling shortened response time and rapid detection of potential threats.
3. Application Security
Application security solutions such as Web Application Firewalls (WAFs) implement ML-based learnings to protect servers and systems against cyber-attacks targeting the application layer (Layer 7) of the OSI model. For example, ML-based solutions can be trained to detect anomalies in HTTP requests and create alarms in case of an attack. They can also be trained to classify the type of attacks (SQL injection, XSS attack) and detect attack vectors. SOC teams can utilize the information coming from these algorithms to fortify cyber defense structures.
4. Authentication Security
In order to prevent cyber identity fraud and account takeovers, there exist many authentication security protocols such as 2FA (two-factor authentication), CAPTCHA (Completely Automated Public Turing test to tell Computers and Humans Apart), or facial and fingerprint recognition.
ML and AI also come into play in authentication security. Facial and fingerprint recognition software directly utilizes ML algorithms to operate, bringing additional protection to hardware systems such as phones and laptops.
ML in authentication security also utilizes other algorithms to better prevent account takeover attacks. Adversaries launch credential stuffing or brute force attacks to access accounts, which may result in breaches in the company network. Through implementing ML algorithms, these attacks can be detected and prevented. The trained system can notice and stop these attacks’ trial and error phase.
5. Attack Surface Management
Managing and monitoring the attack surface of an organization can be a hassle for SOC teams since the digital attack surface is continuously growing for large companies. An AI-based approach to attack surface management can take the burden off SOC teams and ensure fault-free management and monitoring.
SOCRadar utilizes ML algorithms to continuously monitor the attack surface of your organization and proactively deliver alarms for vulnerabilities on your attack surface (e.g., Critical Open Port Detected Alert). Below, you can see an example alert created by SOCRadar’s AttackMapper which utilizes ML algorithms in its operations.
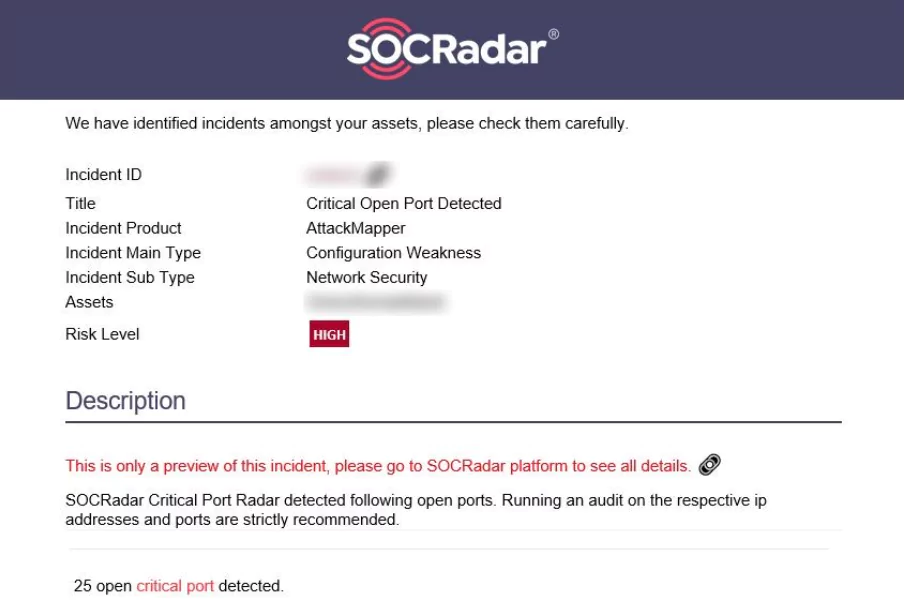
Discover SOCRadar® Free Edition
With SOCRadar® Free Edition, you’ll be able to:
- Discover your unknown hacker-exposed assets
- Check if your IP addresses tagged as malicious
- Monitor your domain name on hacked websites and phishing databases
- Get notified when a critical zero-day vulnerability is disclosed
Free for 12 months for 1 corporate domain and 100 auto-discovered digital assets.
Get free access.